Beating the best with Artificial Intelligence
From millions of data sets to a single click – what’s next for deep learning in SMT?The ability of manufacturing systems to predict and adapt to unforeseen situations, often referred to as Artificial intelligence (AI), promises to revolutionize the electronics assembly market. In a period of just five to ten years, deep learning researchers at Mycronic predict a highly automated SMT environment approaching zero defects with little to no human intervention.
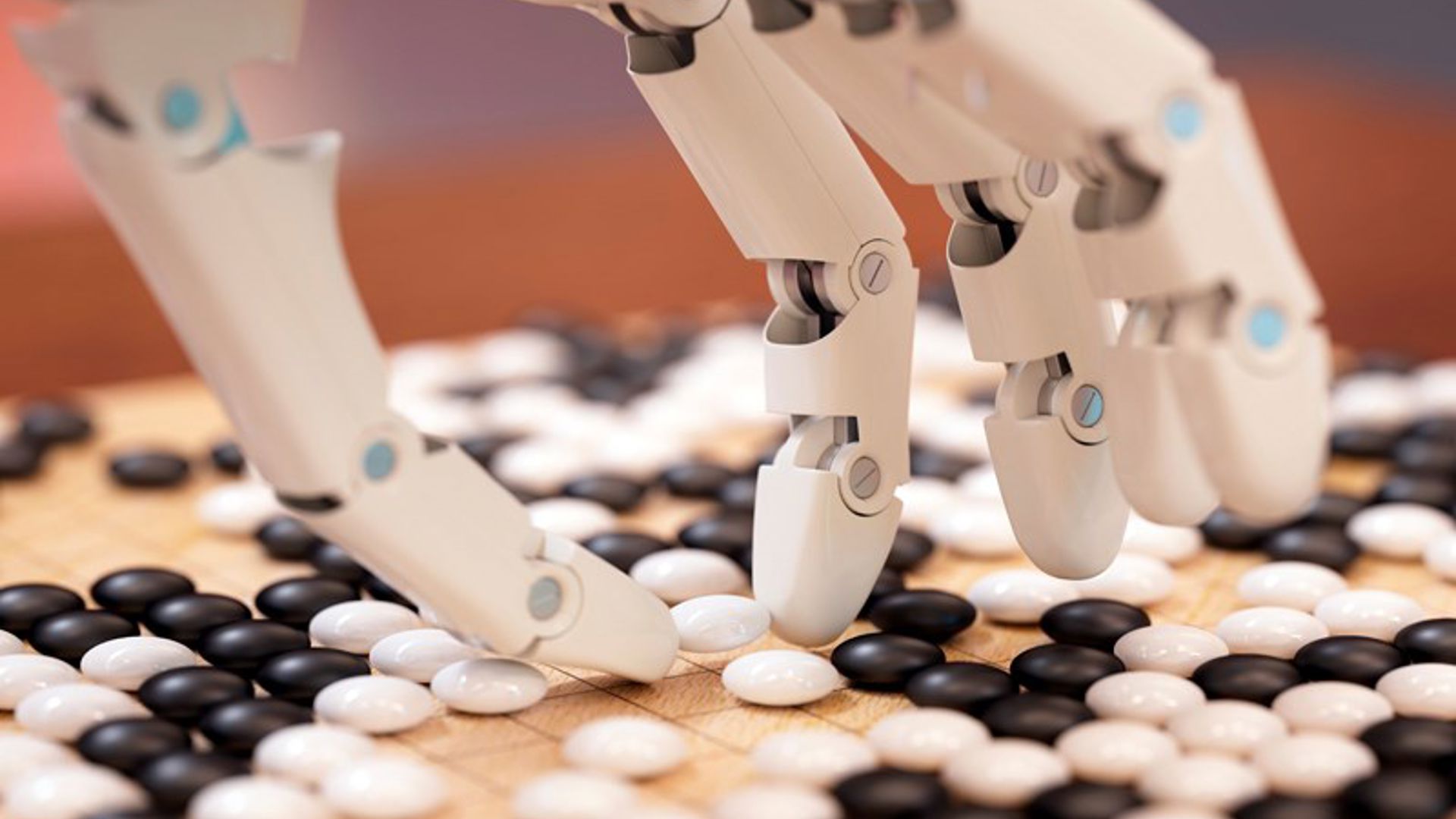
“THIS IS NOT SCIENCE FICTION – WE’RE WELL ON OUR WAY RIGHT NOW,” says Romain Roux, a PhD deep learning engineer at Mycronic’s Center for Deep Learning in Electronics Manufacturing (CDLe) in San Jose, California. The center is currently conducting research into deep learning and AI applications for electronics manufacturing.
Reinforcement Learning – the secret sauce
To illustrate the speed of development in AI, Roux recalls how already in 2017 Google’s DeepMind AlphaGo AI defeated the world’s number-one Go player. Regarded as far more complex than chess or poker, Go is an abstract strategy board game for two players involving black and white stone pieces in which the aim is to surround more territory than the opponent. “The milestone victory by AI was enabled by something called ‘reinforcement learning’ – meaning the use of autonomous new observations and decision-making based on studying previous patterns and playing thousands of games against itself,” says Roux. “At the time, everyone thought this achievement would have taken at least another 20 years!”
From basic Chess to highly complex Go
According to Roux, it is this new level of deep learning and computing power that opens up huge new potential. Returning to the example of Go versus Chess, he notes how an average Go game has 200 moves versus 37 in chess. “ When IBM’s Deep Blue chess-playing computer beat leading chess champions 20 years ago, it could process fewer move possibilities in this closed-loop game.” By contrast, Go involves up to 320 billion possible combinations or more. “Clearly no human doing rulebased programming can handle this. It was AlphaGo’s neural networks that allowed it to creatively sort through millions of data points to develop new strategies and recognize new patterns.”
Vision: the intelligent Mycronic 4.0 factory
Intent on accelerating the development of deep learning and AI for the benefit of its customers, Mycronic expects to incorporate it into our product development roadmaps in 2020. “Our focus is on the Mycronic 4.0 intelligent factory, which relies on factorywide information flows – horizontal, vertical and into the cloud,” he says. “Our ambition is the zero-defect line, and we are already gathering data in collaboration with PCB manufacturers at multiple sites.”
Challenge: achieving repeatable accuracy
According to Romain, in addition to gathering large data sets and image libraries, a key hurdle is achieving repeatable and accurate recognition of a component’s geometry. This will mean improving automated optical inspection systems (AOI) to ensure the stability and accuracy of the definition of new components, even in dynamic production environments where designs, assembly fluids and packaging change rapidly. For Mycronic, this has required building up a large library of tens of thousands of 3D images, including data on complex geometries, that can be quickly recognized using algorithmic assistance.
“This is a good start” he says. “We will need ten times or even a hundred times more data so that our deep neural network can generalize to all types of components and all types of boards. This amount of data is quite usual for deep learning applications”.
He explains that machine learning – which is necessary for a range of auto-programming, closed-loop and predictive systems for Mycronic equipment today – involves parsing structured data to train machine learning algorithms according to defined criteria. Going a step further, deep learning, a subfield of machine learning and AI, structures the algorithms in layers to create an artificial neural network that can create and simulate new situations in order to improve its decision-making without relying on rule-based programming.
The emergence of Digital Twins
Today, deep learning scientists are building virtual replicas of physical factories and combining this with AI and analytics to simulate operations in real time. Often referred to as Digital Twins, this new approach allows for the analysis of data and systems (in the virtual model) to head off problems before they occur in the physical factory. According to Roux, this will enable predictive maintenance, zero-defect manufacturing and creative solutions to product designs in simulated environments. He estimates that the widespread use of Digital Twins in the SMT industry may be ten years down the road. It will also depend on another key breakthrough: synchronized data correlation of all systems in the different machines – something that is not possible today.
Taking steps in the right direction
Mycronic has already developed MyPro Link, an advanced software suite that gives better control and actionable insights into product quality and process automation. It offers data correlation between SPI and AOIs from the same line, and from several lines, without the need for a Manufacturing Execution System. The system continues to improve with the help of larger sets of quality training data. “The addition of deep learning functionality will take the benefits of this system to a whole new level in the years ahead.”